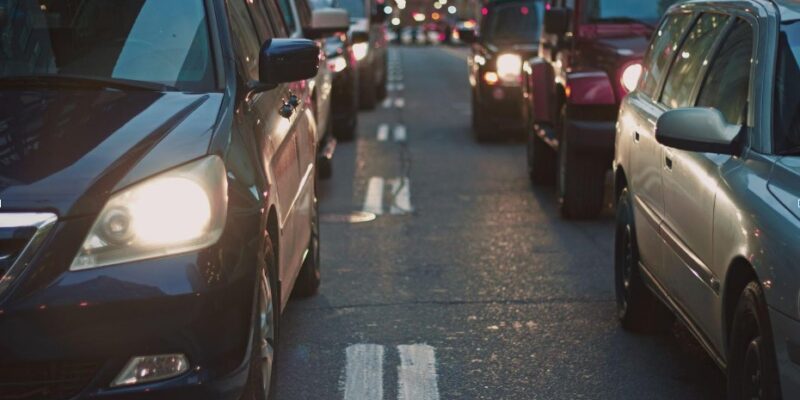
Deep learning techniques have pushed visual recognition to lead the trend in the market. Various industries that staked on automation and AI-driven solutions have adopted computer vision technology across their applications. Healthcare and manufacturing industries were the first movers of computer vision application. They continue to lead the path, together with automotive, agriculture, and security sectors.
Global demand for more efficient digital transformation continues to grow. We need more solutions that will use even larger datasets and offer real-time processing. How can we thus elevate AI capabilities and make computer vision more efficient? What about data annotation, and how does it contribute to the workflow? Let’s see how the first cannot succeed without the second, and how data annotation can directly influence the final results of computer vision.
Current Real-Life Applications of Computer Vision
You’re most likely inclined to security measures at work and face recognition with your smartphone. But these are not the only popular applications of computer vision. Fortune Business Insights reports that in the last year, the most popular computer vision tasks were facial recognition, image classification, and object detection. Where do we see them in our daily lives?
- Surveillance and security. Think of all the cameras installed in crowded places. Security agencies and legal authorities have been using computer vision for years now. Airports, public places, and massive events are only the beginning of the list.
- Social media. We all know that automatic feature when social media offers us to tag a friend on the photo. Their recognition algorithms are based on computer vision techniques.
- Healthcare. Image classification is used massively in medical treatments and diagnosis. It contributes to better identification of diseases, and as a result, more accurate doctor’s prescriptions.
- Retail. Thanks to image classification, you regularly see your favorite stores offering you similar products based on your preferences. The same applies to product discovery and your search functionality.
- Agriculture. Thanks to the expansion of drones and the advancement of AI algorithms, farmers can now proactively detect plant diseases or nutrient deficiencies.
- Autonomous vehicles. If you have already had a ride in a self-driving taxi, you have witnessed the magic of technology. Navigating in the space, omitting pedestrians – all this won’t be possible without computer vision.
- Manufacturing. Mostly used in production lines, computer vision helps to detect defects and monitor quality of produced goods.
We are amazed at how new technology can make us more efficient and productive. New applications appear all the time, and they continually evolve. But what happens backstage? Where does the computer vision journey actually start?
Data Annotation — the Foundation of Successful AI Application
Every AI model starts with data. Tons of datasets are fed into machine learning algorithms as it continually learns. That’s why the more successful the outcome, the more accurate data should be. Given that your raw data come from various sources and in different formats, you cannot avoid addressing one of computer vision services.
The team of experts at Label Your Data can help you with data annotation on every task. Whether you’ll work with face recognition, object detection, or any other computer vision task, data annotation will make your data more accurate and the model’s output more precise. With advanced approach, Label Your Data works with the following types of data annotation:
- Image categorization. This type of data annotation labels the images with different categories. This will further allow machine learning models identifying images per categories.
- Semantic segmentation. This type allows classifying objects in the image per category. With annotated data, a machine learning model will be able to understand the objects in the image (e.g. people, vehicles, buildings).
- 2D boxes. The annotation is done with bounding boxes around an object on the picture. What we frame with it will depend on the task.
- Polygons. With the same function as 2D boxes, polygons define objects, but from different sides. This allows machine learning models to recognize objects with their shape.
- Optical Character Recognition (OCR). This type of annotation deciphers text in the images (e.g. photos, scanned copies) into regular text.
- 3D cuboids. Allow annotating objects with 3-dimensional frames.
- Key Points. This type of annotation puts the most critical points for further shape detection. This is particularly spread in face and body key points detection. A machine learning model will further use it in recognition of poses or emotions.
- Video annotation. This annotation has the functionality of object detection, which is split into frames. As the video plays, the frame moves with the object.
How These Annotation Types Improve AI Models
Every type of annotation corresponds to the task that a machine learning model will perform afterward. Whether the focus is on classification or object detection, with the annotated data, the model will perform better and will give correct results. The precise object detection will help AI models identify where objects are located on the images, what group they belong to. The accuracy is critical in every application.
The differentiation between objects is what will make an AI model understand that the image contains more than one object or differentiate between backgrounds. In videos, AI models will be able to predict movements, analyze actions, and interpret multiple dimensions.
As models become increasingly sophisticated, the role of precise and diverse data annotation in advancing computer vision capabilities continues to grow, enabling more complex, nuanced, and intelligent AI applications across various domains.
Advancing and Progressing
As we look to the future, the role of data annotation in elevating the capabilities of computer vision technologies becomes even more critical. It is the mediator between the digital and the visual. It enables AI systems to process and analyze the visual data with a level of precision that mirrors human perception.
To continue its development, computer vision should go hand in hand with high-quality data annotation. It is this commitment to detailed and accurate data that will push the accurate results of computer vision to the next level. This will in turn bring us new possibilities and applications that will further transform our interaction with technology.